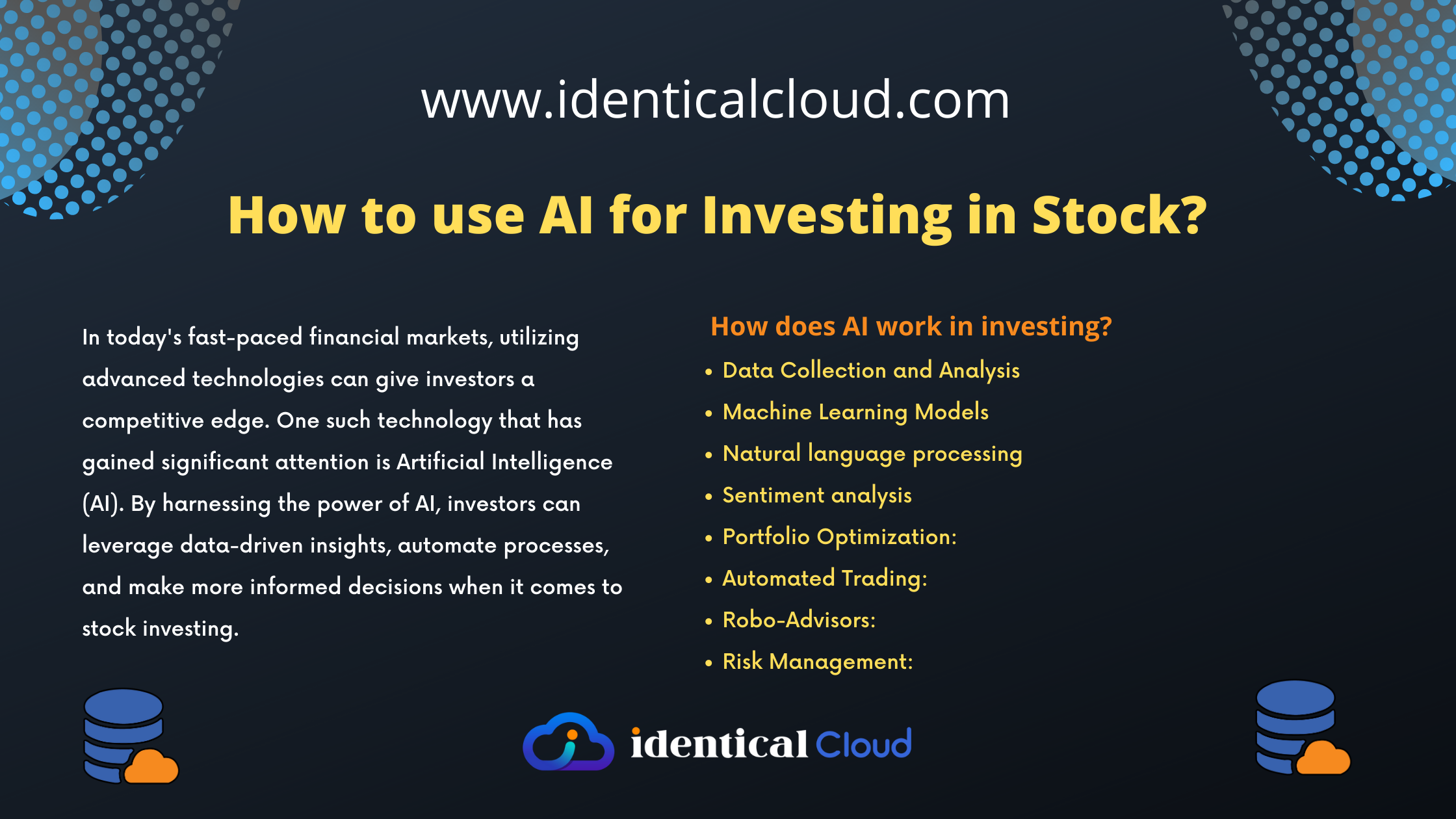
How to use AI for Investing in Stock?
How to use AI for Investing in Stock?
In today’s fast-paced financial markets, utilizing advanced technologies can give investors a competitive edge. One such technology that has gained significant attention is Artificial Intelligence (AI). By harnessing the power of AI, investors can leverage data-driven insights, automate processes, and make more informed decisions when it comes to stock investing.
Artificial intelligence (AI) is rapidly changing the world, and the field of investing is no exception. AI-powered investment platforms are now available that can help investors make more informed decisions about where to put their money.
In this blog, we will explore how to effectively use AI for investing in stocks and maximize opportunities in the market.
How does AI work in investing?
AI-powered investment platforms use a variety of techniques to analyze data and make predictions about the stock market. These techniques include:
1. Data Collection and Analysis:
AI excels at processing vast amounts of data and extracting meaningful patterns. Start by collecting relevant financial data, including historical prices, company financials, news sentiment, and market indicators. AI algorithms can then analyze this data to identify trends, correlations, and anomalies that may impact stock performance.
AI-powered investment platforms collect data from a variety of sources, including:
- Historical stock prices: This data is used to train machine learning algorithms to identify patterns and make predictions about future stock prices.
- News articles: This data is used to identify trends that could affect the stock market, such as new product releases or changes in government policy.
- Social media posts: This data is used to gauge investor sentiment, which can be used to predict how the stock market might react to certain events.
Once the data is collected, it is analyzed using a variety of techniques:
- Machine learning: Machine learning algorithms are trained on historical data to identify patterns and make predictions about future stock prices.
- Natural language processing: Natural language processing (NLP) algorithms are used to analyze news articles and social media posts to identify trends that could affect the stock market.
- Sentiment analysis: Sentiment analysis is used to identify the overall sentiment of a news article or social media post, which can be used to gauge investor sentiment.
The results of the analysis are then used to generate trading signals or to make recommendations about how to invest.
Here are some of the challenges involved in data collection and analysis for AI-powered investment platforms:
- Data quality: The quality of the data is critical to the accuracy of the predictions. If the data is inaccurate or incomplete, the predictions will be unreliable.
- Data volume: The amount of data that needs to be collected and analyzed is constantly growing. This can make it difficult to keep up with the data and to analyze it in a timely manner.
- Data bias: The data used to train machine learning algorithms can be biased, which can lead to inaccurate predictions. It is important to be aware of the potential for bias in the data and to take steps to mitigate it.
Despite these challenges, data collection and analysis are essential for AI-powered investment platforms. By collecting and analyzing data from a variety of sources, these platforms can make more informed predictions about the stock market and help investors make better investment decisions.
2. Machine Learning Models
Machine learning models are used by AI-powered investment platforms to analyze data and make predictions about the stock market. These models are trained on historical data to identify patterns and make predictions about future stock prices.
There are a variety of machine learning models that can be used for stock market prediction, including:
- Linear regression: Linear regression is a simple but effective machine learning model that can be used to predict future stock prices based on historical data.
- Support vector machines: Support vector machines are a more complex machine learning model that can be used to predict future stock prices based on a wider range of features.
- Random forests: Random forests are a type of ensemble model that combines multiple machine learning models to make predictions.
- Deep learning: Deep learning is a powerful machine learning technique that can be used to make predictions about the stock market based on a large amount of data.
The choice of machine learning model depends on the specific application. For example, linear regression may be a good choice for a simple stock market prediction model, while deep learning may be a better choice for a more complex model that needs to take into account a wider range of features.
Here are some of the challenges involved in using machine learning models for stock market prediction:
- Data availability: The availability of data is critical to the accuracy of the predictions. If the data is not available, or if it is not accurate, the predictions will be unreliable.
- Data quality: The quality of the data is also critical to the accuracy of the predictions. If the data is noisy or incomplete, the predictions will be unreliable.
- Model complexity: The complexity of the machine learning model can also affect the accuracy of the predictions. If the model is too complex, it may be overfitting the data and will not be able to make accurate predictions on new data.
Despite these challenges, machine learning models are a powerful tool that can be used to make better investment decisions. By using machine learning models, AI-powered investment platforms can analyze data from a variety of sources and make more informed predictions about the stock market.
3. Natural language processing
Natural language processing (NLP) is a field of computer science that deals with the interaction between computers and human (natural) languages. NLP is used in a variety of applications, including machine translation, text analysis, and sentiment analysis.
In the context of AI-powered investment platforms, NLP is used to analyze news articles and social media posts to identify trends that could affect the stock market. For example, NLP can be used to identify the following:
- New product releases: If a company releases a new product that is well-received by consumers, this could lead to an increase in the stock price of the company.
- Changes in government policy: If the government changes its policy on something like taxes or regulations, this could have a significant impact on the stock market.
- Investor sentiment: NLP can be used to gauge investor sentiment, which can be used to predict how the stock market might react to certain events.
By analyzing news articles and social media posts using NLP, AI-powered investment platforms can get a better understanding of the factors that could affect the stock market. This information can then be used to make more informed investment decisions.
Here are some of the challenges involved in using NLP for stock market prediction:
- Data availability: The availability of data is critical to the accuracy of the predictions. If the data is not available, or if it is not accurate, the predictions will be unreliable.
- Data quality: The quality of the data is also critical to the accuracy of the predictions. If the data is noisy or incomplete, the predictions will be unreliable.
- Model complexity: The complexity of the NLP model can also affect the accuracy of the predictions. If the model is too complex, it may be overfitting the data and will not be able to make accurate predictions on new data.
Despite these challenges, NLP is a powerful tool that can be used to make better investment decisions. By using NLP, AI-powered investment platforms can analyze data from a variety of sources and make more informed predictions about the stock market.
4. Sentiment analysis
Sentiment analysis is a field of natural language processing (NLP) that deals with the identification and extraction of subjective information from text. Sentiment analysis is used in a variety of applications, including customer service, marketing, and finance.
In the context of AI-powered investment platforms, sentiment analysis is used to gauge investor sentiment, which can be used to predict how the stock market might react to certain events. For example, if there is a lot of negative sentiment about a particular stock, this could lead to a decline in the stock price.
There are a number of ways to perform sentiment analysis, including:
- Rule-based sentiment analysis: This approach uses a set of rules to determine the sentiment of a piece of text. For example, a rule might state that the word “happy” indicates positive sentiment, while the word “sad” indicates negative sentiment.
- Machine learning sentiment analysis: This approach uses machine learning algorithms to learn the relationship between words and sentiment. This approach is more accurate than rule-based sentiment analysis, but it requires more data to train the machine learning algorithms.
AI-powered investment platforms can use sentiment analysis to get a better understanding of how investors are feeling about a particular stock or the market as a whole. This information can then be used to make more informed investment decisions.
Here are some of the challenges involved in using sentiment analysis for stock market prediction:
- Data availability: The availability of data is critical to the accuracy of the predictions. If the data is not available, or if it is not accurate, the predictions will be unreliable.
- Data quality: The quality of the data is also critical to the accuracy of the predictions. If the data is noisy or incomplete, the predictions will be unreliable.
- Model complexity: The complexity of the sentiment analysis model can also affect the accuracy of the predictions. If the model is too complex, it may be overfitting the data and will not be able to make accurate predictions on new data.
Despite these challenges, sentiment analysis is a powerful tool that can be used to make better investment decisions. By using sentiment analysis, AI-powered investment platforms can analyze data from a variety of sources and make more informed predictions about the stock market.
5. Portfolio Optimization
Portfolio optimization is a process of selecting a set of assets that will maximize return while minimizing risk. AI-powered investment platforms use portfolio optimization to help investors create portfolios that are tailored to their individual risk tolerance and investment goals.
There are a number of different portfolio optimization techniques that can be used, including:
- Mean-variance optimization: This approach minimizes the variance of a portfolio while maximizing its expected return.
- Modern portfolio theory: This approach takes into account the correlation between assets when optimizing a portfolio.
- Black-Litterman model: This approach uses a Bayesian approach to portfolio optimization.
The choice of portfolio optimization technique depends on the specific application. For example, mean-variance optimization may be a good choice for a simple portfolio, while Black-Litterman model may be a better choice for a more complex portfolio that needs to take into account a wider range of factors.
AI-powered investment platforms can use portfolio optimization to help investors create portfolios that are tailored to their individual risk tolerance and investment goals. For example, an investor who is risk-averse may want to create a portfolio with a low variance, while an investor who is more aggressive may want to create a portfolio with a higher expected return.
Here are some of the challenges involved in using portfolio optimization for investment:
- Data availability: The availability of data is critical to the accuracy of the predictions. If the data is not available, or if it is not accurate, the predictions will be unreliable.
- Data quality: The quality of the data is also critical to the accuracy of the predictions. If the data is noisy or incomplete, the predictions will be unreliable.
- Model complexity: The complexity of the portfolio optimization model can also affect the accuracy of the predictions. If the model is too complex, it may be overfitting the data and will not be able to make accurate predictions on new data.
Despite these challenges, portfolio optimization is a powerful tool that can be used to make better investment decisions. By using portfolio optimization, AI-powered investment platforms can analyze data from a variety of sources and create portfolios that are tailored to the individual needs of their clients.
6. Automated Trading
Automated trading is the use of software to automatically buy and sell assets, such as stocks, bonds, and commodities. AI-powered investment platforms use automated trading to execute trades based on the predictions made by their machine learning models.
There are a number of benefits to using automated trading, including:
- Speed: Automated trading can execute trades much faster than a human trader, which can be an advantage in volatile markets.
- Accuracy: Automated trading can be more accurate than human trading, as it is not subject to human emotions or biases.
- Consistency: Automated trading can be more consistent than human trading, as it is not affected by fatigue or other factors that can affect human decision-making.
However, there are also some risks associated with using automated trading, including:
- Losses: Automated trading can lead to losses if the predictions made by the machine learning models are incorrect.
- Complexity: Automated trading can be complex to set up and manage, and it may not be suitable for all investors.
- Regulation: Automated trading may be subject to regulation, which can add complexity and cost.
Overall, automated trading is a powerful tool that can be used to improve the efficiency and accuracy of investment decisions. However, it is important to be aware of the risks involved before using automated trading.
Here are some of the challenges involved in using automated trading:
- Data availability: The availability of data is critical to the accuracy of the predictions. If the data is not available, or if it is not accurate, the predictions will be unreliable.
- Data quality: The quality of the data is also critical to the accuracy of the predictions. If the data is noisy or incomplete, the predictions will be unreliable.
- Model complexity: The complexity of the automated trading model can also affect the accuracy of the predictions. If the model is too complex, it may be overfitting the data and will not be able to make accurate predictions on new data.
Despite these challenges, automated trading is a powerful tool that can be used to make better investment decisions. By using automated trading, AI-powered investment platforms can analyze data from a variety of sources and execute trades based on the predictions made by their machine learning models.
7. Robo-Advisors:
Robo-advisors are automated investment services that use algorithms to create and manage investment portfolios. They are a type of AI-powered investment platform that can help investors make better investment decisions.
Robo-advisors typically use a combination of machine learning, natural language processing, and portfolio optimization to create and manage investment portfolios. They can also provide investors with personalized advice and guidance.
Here are some of the benefits of using robo-advisors:
- Low cost: Robo-advisors are typically much cheaper than traditional investment advisors.
- Convenience: Robo-advisors can make it easy to invest, even if you don’t have a lot of experience.
- Transparency: Robo-advisors typically provide investors with clear and transparent information about their investments.
However, there are also some risks associated with using robo-advisors:
- Algorithmic bias: Robo-advisors are still under development, and there is a risk of algorithmic bias in their algorithms.
- Lack of human interaction: Some investors may prefer to have more human interaction with their investment advisor.
- Complexity: Robo-advisors can be complex to set up and use, and they may not be suitable for all investors.
Overall, robo-advisors are a powerful tool that can help investors make better investment decisions. However, it is important to be aware of the risks involved before using robo-advisors.
Here are some of the challenges involved in using robo-advisors:
- Data availability: The availability of data is critical to the accuracy of the predictions. If the data is not available, or if it is not accurate, the predictions will be unreliable.
- Data quality: The quality of the data is also critical to the accuracy of the predictions. If the data is noisy or incomplete, the predictions will be unreliable.
- Model complexity: The complexity of the robo-advisor model can also affect the accuracy of the predictions. If the model is too complex, it may be overfitting the data and will not be able to make accurate predictions on new data.
Despite these challenges, robo-advisors are a powerful tool that can be used to make better investment decisions. By using robo-advisors, AI-powered investment platforms can analyze data from a variety of sources and create portfolios that are tailored to the individual needs of their clients.
8. Risk Management:
Risk management is the process of identifying, assessing, and managing risks. It is an essential part of any investment strategy, and it is especially important for AI-powered investment platforms.
There are a number of different risks that AI-powered investment platforms need to manage, including:
- Market risk: This is the risk that the stock market will go down, which could lead to losses for investors.
- Systematic risk: This is the risk that the entire financial system will collapse, which could lead to losses for investors.
- Operational risk: This is the risk of errors or fraud that could occur within the AI-powered investment platform.
- Model risk: This is the risk that the machine learning models used by the AI-powered investment platform are inaccurate.
AI-powered investment platforms can use a variety of techniques to manage risk, including:
- Diversification: This involves investing in a variety of assets, which can help to reduce risk.
- Risk limits: This involves setting limits on the amount of risk that an investor is willing to take.
- Hedging: This involves using financial instruments to protect against losses.
- Monitoring: This involves regularly monitoring the risks associated with an investment portfolio.
By using risk management techniques, AI-powered investment platforms can help to protect investors from losses and ensure that their investments are aligned with their risk tolerance.
Here are some of the challenges involved in risk management for AI-powered investment platforms:
- Data availability: The availability of data is critical to the accuracy of risk management models. If the data is not available, or if it is not accurate, the models will be unreliable.
- Model complexity: The complexity of risk management models can also affect their accuracy. If the models are too complex, they may be overfitting the data and will not be able to make accurate predictions on new data.
- Model biases: Risk management models can be biased, which can lead to inaccurate predictions. It is important to be aware of the potential for bias in the models and to take steps to mitigate it.
Despite these challenges, risk management is an essential part of any AI-powered investment platform. By using risk management techniques, AI-powered investment platforms can help to protect investors from losses and ensure that their investments are aligned with their risk tolerance.
How to use AI for investing
There are a number of ways to use AI for investing. Here are a few examples:
- Use AI to identify undervalued stocks: AI-powered investment platforms can be used to identify stocks that are undervalued based on their historical performance and current market conditions.
- Use AI to generate trading signals: AI-powered investment platforms can be used to generate trading signals based on their analysis of the stock market. These signals can be used to help investors make buy and sell decisions.
- Use AI to manage your portfolio: AI-powered investment platforms can be used to manage your portfolio by automatically rebalancing your portfolio and making adjustments to your asset allocation.
Is AI the future of investing?
AI is still a relatively new technology in the field of investing, but it has the potential to revolutionize the way we invest. As AI algorithms become more sophisticated, they will be able to analyze more data and make more accurate predictions about the stock market. This could lead to better investment decisions and higher returns for investors.
However, there are also some risks associated with using AI for investing. For example, AI algorithms can be biased, and they may not always be able to predict the stock market accurately. Additionally, AI-powered investment platforms can be complex and expensive to use.
Tips for using AI for investing:
- Do your research: Before you start using AI for investing, it is important to do your research and understand how AI works. This will help you make sure that you are using AI in a way that is safe and effective.
- Start small: If you are new to AI investing, it is a good idea to start small. This will allow you to learn how AI works and how to use it without risking too much money.
- Rebalance your portfolio regularly: AI-powered investment platforms can help you rebalance your portfolio regularly. This will help you ensure that your portfolio is always aligned with your investment goals.
- Monitor your investments: It is important to monitor your investments regularly, even if you are using AI to help you make investment decisions. This will help you identify any problems early on and make adjustments to your portfolio as needed.
Overall, AI is a powerful tool that can be used to improve investment decisions. However, it is important to be aware of the risks associated with using AI before you start using it to invest your money.